Clean datasets have similar properties and look the same, while “dirty” datasets are messy in their own ways. Knowing what clean data looks like and how to clean data is an important skill in assisting researchers in making their data FAIR ( findable, accessible, interoperable, and reusable).
In this webinar, you will learn to identify the components of a clean and tidy dataset and describe the steps needed to process a “dirty” dataset. With these components identified, you will be able to tidy your own data and provide guidance to researchers.
You’ll see, in action, common data issues solved by carrying out data transformation and pivoting operations. You’ll also learn the steps needed to break down observational units into separate tables (“normalize” data) so they can be efficiently stored in databases.
This webinar is a companion to Clean & Tidy Data: Getting Started with Spreadsheet Data. The webinars stand alone and work together synergistically. Getting Started will show you best practices for beginning to work with medical data.
This is a required core course for Level II of the Data Services Specialization.
Learning Outcomes
At the end of the webinar, participants will be able to:
- Identify the components of a clean and tidy dataset
- Apply knowledge of the components of a clean and tidy dataset to cleaning data
- Identify the steps of normalizing data
Audience
Medical librarians and other health information professionals who provide or plan to provide data services. Familiarity with browsing and editing spreadsheets is helpful.
Presenters
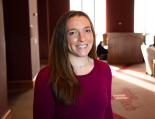
Anne M. Brown is an Assistant Professor in Data Services, University Libraries at Virginia Tech and affiliate faculty member in the Department of Biochemistry and Academy of Integrated Science. She is the author or co-author of a number of publications and presentations on data-related and data literacy topics.
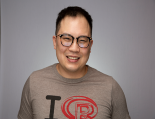
Daniel Chen is a graduate student in Genetics, Bioinformatics, and Computational Biology at Virginia Tech. His research is focused on data science education and pedagogy in the medical and biomedical sciences. He is the author of Pandas for Everyone: Python Data Analysis and a number of other data science learning materials.
Registration Information
- Length: 1.5 hour recorded webinar
- Technical information: After you have registered, go to My Learning in MEDLIB-ED to access the recorded webinar, resources, evaluation, and certificate.
- Register, participate, and earn 1.5 MLA continuing education (CE) contact hours.